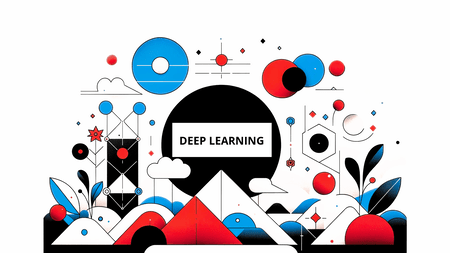
Deep learning: a key concept of modern AI
Deep learning has made considerable progress in recent years. This explanation of technical terms gives you a comprehensive and easy-to-understand introduction to this fascinating topic.
Short and sweet:
- What is deep learning? An advanced method of machine learning based on neural networks.
- Applications: From speech recognition to image analysis.
- Challenges: Data requirements, computing power and ethical considerations.
What is deep learning?
Deep learning is a subset of machine learning that is characterized by the use of artificial neural networks. These networks are inspired by the human brain and consist of layers of neurons that can recognize complex patterns in data.
Basics of deep learning
- Neural networks: Consist of input, hidden and output layers.
- Learning process: The network learns from data by adjusting weights.
- Algorithms: Different algorithms are used to accomplish specific tasks.
Use of deep learning
Deep learning is used in a variety of areas, including
- Speech recognition: systems such as Siri or Google Assistant.
- Image recognition: From facial recognition to medical diagnostics.
- Automated decisions: In areas such as finance and transportation systems.
Deep learning in practice
Examples of successful applications of deep learning include
- Self-driving cars: using deep learning to process traffic data.
- Personalized advertising: Use in online marketing for target group analysis.
Challenges and limitations
Despite its progress, deep learning faces challenges such as
- Data requirements: Requires large amounts of data for effective learning.
- Computing power: High demand for computing capacity.
- Ethics: Data protection and AI ethics issues.
The future of deep learning
The future of deep learning opens up a kaleidoscope of promising opportunities and ground-breaking developments in a variety of areas.
Innovative improvements in algorithms.
This vision of the future includes the creation of advanced algorithms that enable even more efficient and faster learning. Such improvements will aim to optimize learning processes to achieve more accurate and reliable results in less time. The advanced algorithms could also help to overcome existing challenges in data processing and interpretation, pushing the boundaries of what can be achieved through machine learning.
Expanding the areas of application
Deep learning will no longer be limited to traditional fields, but will expand its horizons to find application in a plethora of new and exciting areas. From robotics, where it will contribute to the development of smarter, autonomous machines, to environmental sciences, where it will be used to model complex ecological systems and predict environmental change. This broadening of application areas will allow complex problems to be solved in different sectors and open up new ways to improve our daily lives and protect our planet.
Overall, a future is emerging in which deep learning will play a key role in advancing technology and promoting human progress.
Further information
We think deep learning remains a dynamic and rapidly evolving field that is constantly pushing the boundaries of what is possible with artificial intelligence. It's exciting to see how deep learning is revolutionizing not only the technology, but also the way we do business and interact with customers.
Further links
FAQ's
Was unterscheidet Deep Learning von traditionellem maschinellem Lernen?
Deep Learning und traditionelles maschinelles Lernen sind beides wichtige Bereiche der künstlichen Intelligenz, aber sie unterscheiden sich in mehreren Schlüsselaspekten. Der Hauptunterschied liegt in der Art und Weise, wie Daten verarbeitet und interpretiert werden. Deep Learning nutzt tiefere (d.h., mehrschichtige) neuronale Netze, die es ermöglichen, komplexere Muster und Beziehungen in den Daten zu erkennen. Diese tiefen Netzwerke bestehen aus mehreren Ebenen, die jeweils darauf trainiert sind, spezifische Merkmale zu erkennen und zu interpretieren. Dadurch kann Deep Learning Aufgaben bewältigen, die für traditionelle maschinelle Lernmethoden zu komplex sind, wie z.B. die Erkennung von Objekten in Bildern oder die Verarbeitung natürlicher Sprache.
Deep Learning nutzt tiefere neuronale Netze und kann komplexere Muster erkennen. Ist Deep Learning immer die beste Wahl?
Trotz seiner fortschrittlichen Fähigkeiten ist Deep Learning jedoch nicht immer die beste Wahl für jedes Problem. Die Effektivität von Deep Learning hängt stark von der Menge und Qualität der verfügbaren Daten ab. Für kleinere oder weniger komplexe Datensätze kann traditionelles maschinelles Lernen effizienter und effektiver sein. Zudem erfordert Deep Learning in der Regel eine höhere Rechenleistung und mehr Ressourcen, was es für kleinere Projekte oder Organisationen mit begrenzten Ressourcen weniger praktikabel macht.
Wie beeinflusst Deep Learning den Online-Marketing-Bereich?
Im Bereich des Online-Marketings hat Deep Learning einen signifikanten Einfluss. Durch die Fähigkeit, große Mengen an Daten zu analysieren und komplexe Muster zu erkennen, ermöglicht Deep Learning präzisere Zielgruppenanalysen und personalisierte Werbung. Unternehmen können mithilfe von Deep Learning-Algorithmen das Verhalten und die Vorlieben ihrer Kunden besser verstehen und ihre Marketingstrategien entsprechend anpassen. Dies führt zu effektiveren Werbekampagnen, da Werbung gezielter und relevanter für den einzelnen Nutzer gestaltet werden kann. Darüber hinaus kann Deep Learning in der Vorhersage von Verbrauchertrends und im Verhaltens-Targeting eingesetzt werden, was zu einer Optimierung der Kundenansprache und einer Steigerung der Konversionsraten führt.